

The basic steps for cleaning data are as follows: For example, if you want to remove trailing spaces, you can create a new column to clean the data by using a formula, filling down the new column, converting that new column's formulas to values, and then removing the original column. Or, if you want to remove duplicate rows, you can quickly do this by using the Remove Duplicates dialog box.Īt other times, you may need to manipulate one or more columns by using a formula to convert the imported values into new values. For example, you can easily use Spell Checker to clean up misspelled words in columns that contain comments or descriptions. Sometimes, the task is straightforward and there is a specific feature that does the job for you. Fortunately, Excel has many features to help you get data in the precise format that you want. Before you can analyze the data, you often need to clean it up. There's a great tutorial for spacy on their website.You don't always have control over the format and type of data that you import from an external data source, such as a database, text file, or a Web page. I recommend playing around with your own dummy data, trying different regular expressions with the re module, and playing around with the wordcloud, spacy and seaborn modules. be removed/replaced or are they a useful predictor? Will removing punctuation improve or reduce a machine learning model's performance or make no difference at all? Should the text be converted to lower case? There's no right answer, so its useful to be able to easily play around with the text data and experiment. Data cleaning and analysis is a big part of working with text data, and deciding what to change, and how, will depend on the problem being solved and is part of the art of data science. are there any empty documents (tweets)? Our dataset is so small that we can see that there aren't any empty tweets but in real data sets that are larger you'd need to find out programmatically.
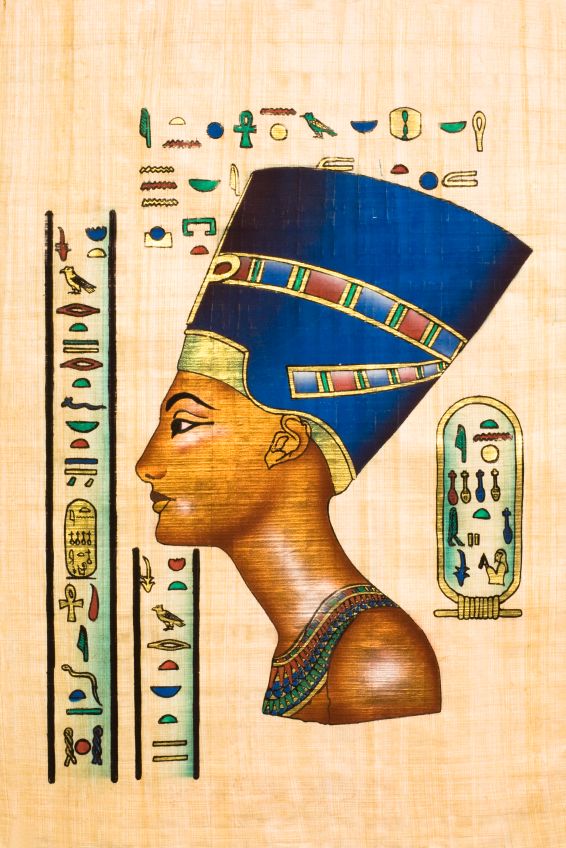
what's the mean average number of tokens? (Answers to these length questions are useful later on if you're going to use machine learning models).how many tokens (words) are in the longest tweet?.There's lots more you can do of course, for example: The chart now gives us a much better indication of the topics being discussed in the tweet text. title ( "Top 25 Most Frequent Words (Excluding Stopwords)" ) plt. sort_values ( by = "freq", ascending = False ). # frequencies (which will exclude the stopwords this time)įig, ax = plt. columns = # display a bar chart showing the top 25 words and their reset_index () # rename the columns to "word" and "freq"įreq_df. from_dict ( tweet_word_freq, orient = 'index' ). Tweet_word_freq = Counter ( tweet_words ) # re-create the Pandas dataframe containing theįreq_df = pd. is_stop != True ] # get the frequency of each word (token) in the tweet string
